.png)
Authors: Kate Glazebrook and Khyati Sundaram
What? A hiring tool that doesn’t use AI to make hiring decisions?!
It’s a sign of the times that one of the most surprising things people discover about Applied is that we don’t use AI to make selection decisions in hiring (for example, see this article in Techcrunch).
This is because more often than not, using AI to make selection decisions entrenches rather than reduces inequalities. And also because we believe that a more collaborative hiring process results in more inclusion. This is why we don’t use AI to decide if you get the job, but we do use data science to help other aspects of hiring.
But let’s start with the selection question.
What to look for in a hiring assessment
When you speak to hiring managers and recruiters about what they want out of a hiring process it’s not unlike what all of us ever want: a perfect multicoloured unicorn. And rightly so: talent is usually the most valuable resource of any organisation, so deciding who to hire is hugely consequential.
Some of the goals people have when they’re deciding how to assess candidates is summarised in Figure 1 below:
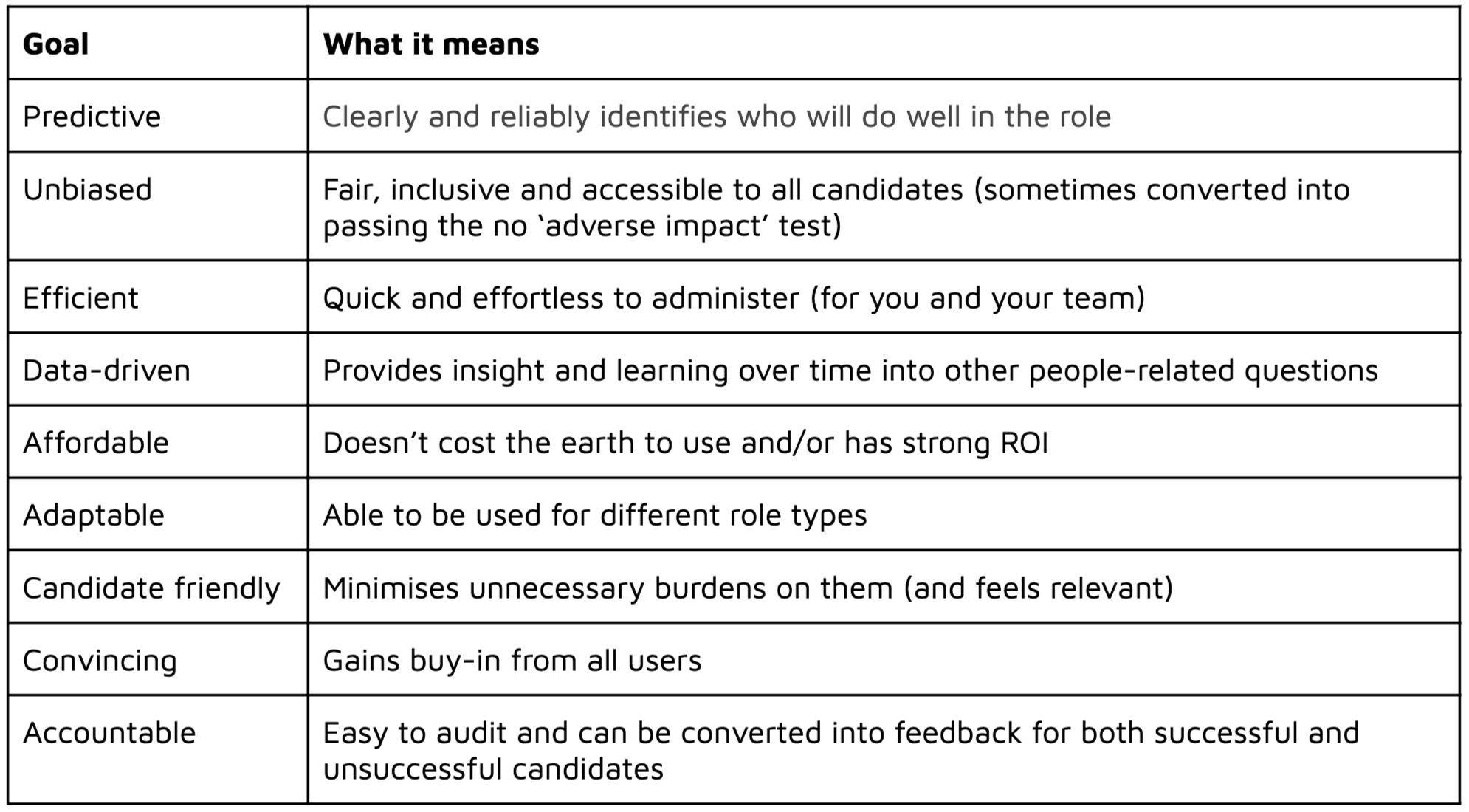
That’s a long and hard list of objectives to balance.
In that context, AI is alluring: its sheer computational power offers the opportunity to ingest infinitely more information than humans could and do it in the proverbial click of a button. So there’s no doubt that once an AI is built (which can take time), the automation it enables saves time. And when most people have a to-do list that’s permanently longer than their arm, that efficiency gain is real.
A well-designed AI can also offer some degree of adaptability (though it relies on very large data-sets to achieve it), and they can be affordable.
So already it’s easy to see why there’s been a massive rise in the development and use of AI in hiring decisions in recent years.
But it doesn’t come cost-free.
Technical challenges
The first - and most common - criticism waged at AI relates to how they’re developed and the risk that AI tools exacerbate inequalities. To understand why, it’s worth doing a very simplistic tour of how they work.
How AI works
The purpose of most AI tools is to predict an outcome so that people can direct resources more effectively - whether that’s hiring teams deciding who to interview, banks deciding who to offer loans to, or social care workers deciding which cases to prioritise.
To do that, the AI seeks to understand the world and make recommendations based on the patterns it sees. Typically, how AI developers achieve this is by identifying large troves of pre-existing data which contain the outcomes they’re looking for (i.e. the characteristics of candidates who got hired, and who did well in the job).
They then split that data into two: the part of the data they build from and the part they use for testing. With the former, they give the AI a goal to achieve and let the algorithm identify the most efficient and accurate path toward that goal. In hiring that would be like saying “given we hired these 50 people, tell us what the characteristics are of those candidates that best separates them from all the people we didn’t hire”.
To measure the effectiveness of the AI, they would test how well their algorithm predicted the outcomes they saw in the second half of the dataset: the greater the level of accuracy, the better.
Why it can be problematic
This process of using ‘training data’ to build future recommendations is often where the issues lie, since that data is not objective. So that means, there was a lot of human judgement and decision-making (intentional or otherwise) that went into the shape of the data, including (but not limited to) the way it was collected, who and what was included in the dataset, the purpose it was originally collected for, and the parameters it contains all have massive impacts on how good the AI using it can be.
As is detailed in books including Made by Humans by Ellen Broad, Weapons of Math Destruction by Cathy O’Neil, and Algorithms of Oppression by Safiya Umoja Noble, articles like this one by Solon Barocas and Andrew Selbst, the work of Joy Buolamwini through the Algorithmic Justice League and many others, these seemingly esoteric statistical points can have huge consequences.
Let’s take just two angles on this: who is included in your data and how it’s classified.
Not including a sufficiently diverse set of underlying people (i.e. by gender, age, ethnic background) can result in algorithms that are effectively imbalanced. With fewer data points on these groups, the algorithms are less accurate in making predictions about them, and this can result in poorer outcomes - ranging from the offensive to the life-changing (as has been shown for many facial recognition algorithms). And it’s probably no surprise that in virtually all cases so far tested, these failures fall disproportionately on underrepresented and underserved populations.
But it’s not just that not having a comprehensive dataset that you have to be worried about, it’s also how that dataset is classified and tagged.
Take for example how most hiring AI tools approach the problem. In order to build an AI that will predict which candidates will be top performers for your organisation, the AI designer first needs to know what a top performer at your organisation looks like. What are the characteristics of those people? What makes them unique?
To ascertain that, they would extract your existing performance data - i.e. the last few years’ worth of performance appraisals, or perhaps they’d use markers like how quickly someone was promoted as a signifier of their quality. They then set the algorithm to identify what unifies those top performers and what sets them apart from the others. With that capacity to differentiate, they then inject candidate data, using the algorithm to identify strong matches.
Many of you will see the challenge built in here: how was performance evaluated? What processes were used to define promotions?
Given that a wealth of behavioural research has identified the biases that lie within the ways we evaluate others (including, for example that access to stretch opportunities and large client accounts, and rapport-building with senior managers are more likely to go to men or white employees - see Iris Bohnet’s book What Works: Gender Equality by Design for a through review) - there is good reason to question the objectivity of the very outcome we’re seeking to automate. Indeed, that’s often what we see - as examples such as women being served up lower paying jobs, women and people of colour being shown different jobs and housing opportunities or Amazon’s hiring AI rejecting women are all too obvious illustrations. (Kate has discussed these and other challenges in an address to the Behavioural Exchange 2019, available here).
And the result is often that AI tools like these literally encode - and reinforce - the bias better than a human could. Or another way of thinking about it is this: an AI will do a great job of recognising and replicating the outcomes we already see, which is problematic given that what we see is systematically biased.
These and many other technical limitations of the ways that AI tools are being developed are being investigated by regulators (such as the recent report by the UK Government’s Centre for Data Ethics and Innovation), civil society organisations (for example the AI Now Institute), and academics (for example, the recent research paper by Jon Kleinberg, Jens Ludwig, Sendhil Mullaination, and Cass Sunstein).
And there is some amazing work being done to develop independent auditing tools (see for example Themis created by academics at the University of Massachusetts, Amhurst) that can identify causal bias in algorithms, which can then be used to help AI developers to improve their algorithms and, ideally, create a standard for what is or isn’t allowed to be used in the real world.
So we have myriads of technical limitations but we also have a problem of gaming the dataset.
If there exists an algorithm, quite often there will be a mechanism that can be used to game the algorithm. If an algorithm has been based on keywords, then it can very easily be manipulated to ‘cheat’ the system. This is one of the key reasons why we at Applied don’t use AI when marking answers in our selection process (you can read more about how Applied works here).
Why not using AI is also better for inclusion and belonging
The second reason we don’t use AI to decide who gets a job relates to inclusion and belonging. Our mission is to help people see talent differently. To uncover that the right person for the job might be someone who looks, sounds, and comes with a personal and professional background that bucks their expectations.
But for that right person to be successful in the job, our work isn’t done when they get hired.
It’s now pretty well accepted that we experience workplaces differently. Being the only woman/person of colour/person with a disability in a workplace will on its own make your experience of it, and how people relate to you, different from everyone else. And a stack of research (again, see Bohnet’s book) proves that to be true: it can unconsciously shape how a manager hands out work opportunities, evaluates your performance, or how they build (or don’t) rapport with you. All of these things can result in equally qualified candidates having unequal opportunities to shine.
As a product that wants to genuinely change the world of work, we feel we have a responsibility to do more than just help with who you choose to hire. While we aren’t present in all of those interactions, we do feel we have an opportunity to frame how both parties feel about the hiring process, which then makes the chances of a positive onboarding and working relationship far higher.
By actively involving team members - including the potential hire’s new manager and the wider team - in making the decision on who their next colleague should be (by scoring candidates themselves, but guard-railing the process against bias), we implicitly build buy-in and ownership over the collective decision.
This matters because it directly targets a concept researchers term ‘algorithm aversion’ which is essentially our tendency to reject decisions taken by computers that we believe we’d be better judges of (even when that’s untrue).
We all believe we’re great judges of other people, which is why we over-rely on unstructured interviews. So if an algorithm tells us the best person we should hire is X, and we don’t like X, we reject the outcome. This could manifest itself in a manager never really spending the time to help a new team member onboard well, which results in them ramping up slower and suffering as a result.
But what the research has also shown is we can mitigate that aversion by allowing people to feel they have some hand in creating or controlling it. They’ve found, for example, allowing people to make some choices in how the process is done significantly improves the chances that people will go with the recommendation it makes. It’s a version of what behavioural scientists have referred to as the ‘Ikea effect’, where we tend to value things more if we’ve had a hand in making them.
So a process that involves humans - but where the decisions taken are protected from bias - ensures that you find the best qualified person for the job. And the successful candidate walks into the job knowing not only that they were the best, but crucially, their new team knows it too, and feels a sense of ownership to make them successful.
But that doesn’t mean we don’t see loads of opportunity to use data science techniques for other aspects of hiring
So we have chosen not to use AI to define who does or doesn’t get a job.
But we do see enormous opportunity for AI and other data science techniques to improve hiring and inclusion, and in particular, to help save time and provide timely nudges.
For example, we have embedded some simple algorithms into our job description analysis tool to help people to write more inclusively and avoid language that might put off candidates from different backgrounds.
We also use data science tools to identify which skills a hiring manager might be hiring for, and make suggestions on the best questions to ask in a hiring assessment from the 1 million+ data points we have accumulated.
We’re also always experimenting with ways to make sure people’s time is used most effectively in the hiring process - currently we’re evaluating AI assisted techniques for such projects.
Such tools should be used to enable hiring teams to do their jobs more effectively, not about deciding who gets the job. And when it comes to AI, that distinction is key.
Find out how we're changing the way the world hires via our resources, or see how it all works for yourself and start a free trial of the Applied platform.